Where Is The Logic In Our Reasoning?
- ehrmaneli
- Jul 28, 2022
- 10 min read
Do “words”, in today’s Computer Natural Language Processing (NLP) systems, denote? To “denote” sounds too specific. Perhaps all that they do is associate. Association is a weighted similarity score to uses of the same word and other words in both other sentences and in labels of images. The resulting list of “neighbors” is not usually even repeatable. Yes, the associations shift, as Derrida objected. But they don’t just shift across time, they shift in every sentence, in every mention. Also, they aren’t defined in terms of other words, there is only a similarity connection (using similarity in its technical NLP understanding)1.
What does that mean for reason and argumentation?
First of all, it means that the basic requirement of logic that two signs written identically can be substituted is hopelessly violated by every text we have ever thought, said or written.
Despite the initial hopelessness that this situation suggests, this article seeks to propose a possible mechanism whereby reason can succeed nevertheless.
We use our concepts and terms in horribly loose and vague application. However, despite our muddled and unstable productions, we can write down a set of assertions. We use the associations of our memories, images, internal categories and semiotic beliefs to write down our assumptions, reasoning and conclusions in words2.
Now that there is an objective text, we can make a profound transition away from the words as understood, meant and emotionally assented to. We now consider the words as written down. These words can be seen as a set of elements from a specific dictionary and arranged as well-formed-formulae (wffs). Yes, each of these words and their arrangement will have different semantics and degrees of emotional assent for each and every reader. Nevertheless, we ignore that and focus on the words as if they have absolutely no semantics; only syntax.
We have created a mapping from the language as used in a putatively reasoned argument onto a set of wffs devoid of any meaning written in a formal language (that may look like English).
Take an example. I write: “(1) Elephants are larger than cats and cats are larger than microbes. (2) Elephants, cats and microbes are objects. (3) For all objects, if X, Y and Z are objects, then if X is larger than Y and Y is larger than Z, then X is larger than Z.”
All the metaphysics in the world about real objects and categories or how we can point to and pick out an object from its background in the image no longer matter once the mapping process of writing down has taken place.
You can feed your text into a formal procedure. You can even imagine writing a computer program. Version 1.0 of this program need only replace strings like “X”, “Y” or “Z” with any constants such as “elephants”, “cats” and “microbes” if there is an assertion such as (2) whose last two words match the two words following “X, Y and Z” in assertion (3). (Namely, the words “are objects” in the example given.)
It might never have crossed your mind that Elephants are larger than microbes. However, the next version of your program, version 2.0, might inform you that Elephants are indeed larger than microbes. In other words, it would not only be able to verify implications that you put in, but it might be able to search for new sentences that are implied by your text. The implied facts were, in a sense, always “there” in what you had said. Which, of course, makes it all the more surprising that you had noticed them.
On the other hand, if you had tacked on to the original text something like: “(4) Elephants are not larger than microbes”, it could have informed you that your reasoning has failed.
What if you had left out assertion (3)? Perhaps we could continue developing the program so that version 3.0 looks for (3) and could tell you that, should you assent to it and have it included, then the text could be verified. Otherwise, no implication can be made. In other words, you just say that these things are objects, but your program is aware of assertions about objects, assertions you might not have considered, and it asks you whether you would assent to them too. Perhaps, by asserting that Elephants are larger than microbes, you had obviously used (3) but since it is common knowledge you had neglected to mention it. That will work when talking to people, but will fail with a simple variable substitution procedure like the one we have here.
Is this what we approximate when we reason or provide arguments? Is the possibility of such mappings, an explanation for why there is any point in continuing to pretend any semblance of rationality when, in fact, our words are so hopelessly fuzzy, slippery, ever-morphing and subjective; when our assertions operate inside our head as no more than loose keys to searches that depend entirely on the contents of the brain in each head and are always biased to whatever priming activated whatever set of neural circuits?
Is this the explanation for why logic, despite everything, can actually explain some of the most amazing achievements of the human enterprise?
If so, there are a number of interesting considerations to point out. These will ultimately lead to a proposal for how this insight can be used to build a good AI.
Note that the text in the example was very simple. The ideas of Saussure and Derrida might suggest that every word requires either an infinite regress of other words or a contrast with all other words in order to provide its meaning. This was not necessary in the simple example given earlier. More complex examples may require many more assertions but not all that many. Only enough assertions such that the symbol replacing procedure can begin. Each of the assertions must be assented to by a particular thinker, system or theory for the reasoning procedure to be important to those thinkers. If they do not assent, then they might decide they need to, or they might simply not care what the logical outcome of any procedure might produce.
There is no need for a philosophical “foundation” to be built for the assertions. Even Quine’s “Web of Beliefs” can be pared down in the extreme to just a set of assertions assented to and selected for this one thesis. That does not mean that for the entire range of interests that a person might have, we might not need to consider the complete web, the change costs associated with each belief and the consistency of the whole. The focus here is only on the process of reason as applied to a selected thesis.
Next, consider that for larger and more complex sets of assertions, the ability to provide an implication requires exponentially more computer resources. As the number of assertions grows, the number of different combinations of word replacements, grows, well, combinatorially.
That said, finding implications is only one kind of task that we could implement. Assume that we want to describe a game played on a grid or chessboard. We label each position and painstakingly describe that, say, “a1 is left of b1” etc. We could write a program that will figure out for you all the steps of how to get a King from b2 to e8. Assume for now you could never have figured that out. Such a program could be written even if the challenge include other pieces that block the simplest path.
A simple way to do that would be to exhaustively search all the positions and all the moves the King could make. We can do better using a technique called Reinforcement Learning (RL). RL would not have to try out all moves, which could end up taking some time. It could focus only on those that are likely to be required, thus reducing the amount of searching. Deep Learning could also be combined with RL to produce results that are better than any human can achieve once the problems presented become really difficult. That said, getting to this level requires a lot of money to pay for the computers and energy needed to beat the human mind. Moreover, this is a field where computers excel. There are others, such as the creation of human prose, where fantastic costs are required – and the computer is still a poor replica of what humans can do.
Also note that the example given would have been just as valid if we replace the word “larger” with non-sense such as “fooier”. Moreover, the constants “Elephant” etc. that were used in the example might be replaced with “boogle”, “timble” and “quank”. That is what we mean by mapping to a semantics-free set of assertions.
This leads to an important conclusion. The same processing that is applied to one problem, can be equally applied to another - as long as some critical type of similarity is maintained. You can replace all the constants and you can replace all the operators (words like “larger”).Critically, for the similarity to hold, the objects and operators must maintain exactly the same relationship to each other. You could call this the same “structure”. Structuralists, in fact, see all of human discourse in terms of structure.
Assume you have two totally unrelated fields. Assume you create a set of semantic-free assertions for two theses, one from each field. As long as the objects and operators maintain the same relations to each other, the results from one thesis in one field can be applied “as is” to the second thesis from a totally unrelated field. An obvious example of two fields might be billiard balls on the one hand and the micro-structure of all matter on the other. Atoms are not billiard balls and they don’t “move” or “bump into each other” in the same sense as billiard balls do. Nevertheless, results from one field can be applied to the other (until some new observations start upending the similarities).
Think back to the example of the chess board mentioned earlier. The point to bear in mind, is that the program would not care that you are describing a chessboard or chess challenges. As far as it is concerned, all the text you give is simply a set of meaningless symbols.
Assume that task 1 is to get the King from a2 to e8. Task 2 is to get the king from g3 to a7. Searching all the possible moves in terms of positions and finding the best path for task 1 takes time. Moreover, all the results for task 1 are of no use for task 2. So you have to start all over again. Now imagine you have a library of functions. You search the library and you find that if you translate the state at any one time into terms such as “left of the target”, “below the target”, not only is your search faster, the same results you found after so much effort for task 1, will apply to task 2 immediately. Of course, searching for functions that return new operators such as “left of” takes even more time. However, once you found it, you can apply it to all the tasks.
Now imagine that you have an entirely different field of activity. Nothing to do with chess and chessboards. However, the same structures hold. You realize that the same kind of switch from absolute positions to relationships between positions will apply in the new field! You instantly have a sophisticated paradigm for solving problems in the new fields.
I suggest that we do this all the time. We are very good at taking the hard-won (or taught) insights and strategies from one set of problems and effortlessly switching the constants and operators so that we can apply similar insights to different problems.
Let’s say I play a computer game called “Starcraft”. Roughly, it involves building factories that then make armies and you send the army to go smash your opponent. Say, I played this game for days. Each time I play, at some point, my computer opponent turns up at my factories with an army and tries damaging my factories. Sometimes I manage to fend off the attack. But a short while later a more powerful army turns up and eventually I get all beaten up. One day, I finally realize that the best time to retaliate is right after I managed to fend off an attack. I send my army and when I get to his factories, I find that he has not had a chance to build more armies. So I destroy his factories and win the game.
It took me a long time to figure out this strategy. But then it turns out that this idea of retaliating right after a successful defense works for lots of other games, even if they seem quite difference from Starcraft.
This transferring of a hard-found solution to a different problem comes naturally to human minds.
You might object that the example is too narrow; that all the games are really the same, or at most there is a designed similarity between some games and some real-world training whether military or economic.
Therefore, consider a child playing with Lego(TM) blocks. The child plays for years with these blocks and develops skills and strategies. What could be further from this experience then the adult pass-time of presenting arguments in support of an intellectual position. It does not take much effort, though, to realize that the core structure of many of the Lego skills can be applied to building and supporting reasoned arguments.
This ability to transfer solutions, strategies or resolution of sub-goals needs to be built into AI. AIs have immense resources for solving specific problems particularly when it can be presented in millions of different forms (training sets). We do not require that much data. We naturally transfer solutions. The key is seeing that the structure of the objects and operators in one field carries significant resemblance to those of another.
In Computer Science terms, the space of combinations in many fields is immense, even considering the speed with which computers can operate. It is theoretically possible, but very wasteful, to search that space for good solutions in each and every case. Moreover, while some fields of enterprise allow for large data collection, others, by their nature, do not.
Teaching computers to focus on the similarity of the syntactical elements in different fields, should therefore be a very productive way for AI to move toward the decades-old challenge; creating a General Problem Solver.
In conclusion, there is one more question that may be asked. Referring back to the problem at the beginning of this article, the impression that words are hopelessly loose, shifting and lacking any meaning that would be stable enough to participate in logical reasoning. The question is, could we not have evolved a better tool for thinking than words and language as it is today.
Purely as speculation, the following answer is proposed. It is exactly the loose nature of words and the tendency for them to apply to so many different situations that gives them a special power. The fact that the same word may be used in one scenario as it might in a different scenario, is what leads the thinker to unknowingly transfer solutions from the first case to the second. This may be true for similar context with differences that we ignore, or for fairly diverse contexts that are only bound by the almost-accidental application of a similar verb or adjective.
It is the very vagueness in our use of words that is both our downfall and the substrate that leads us to this awesome capability to structural transference.
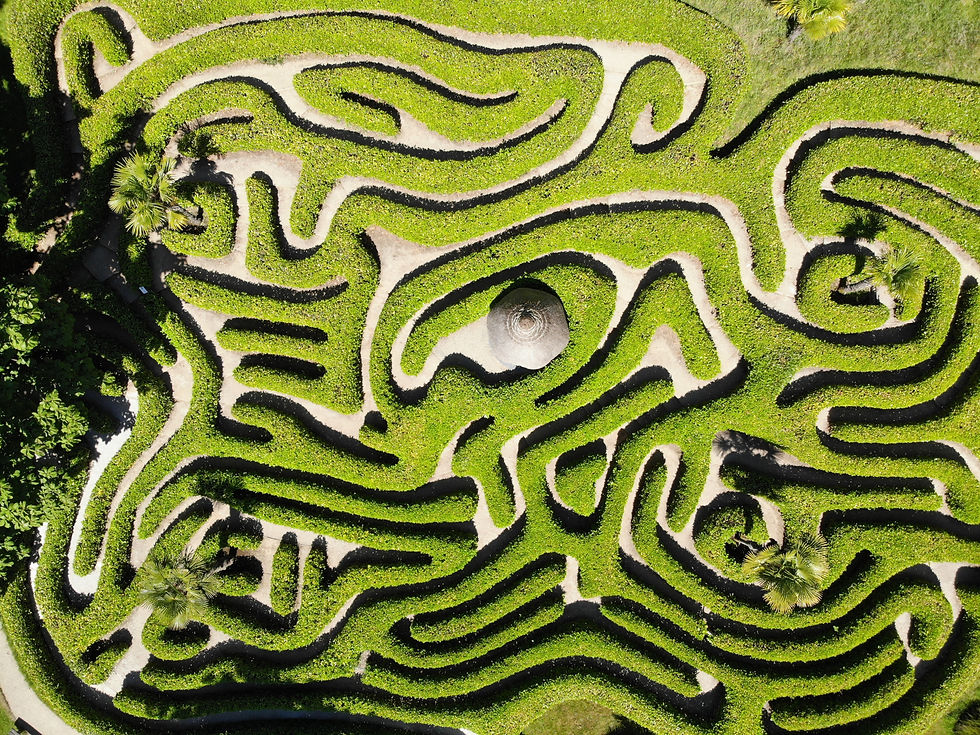
Photo by Benjamin Elliott on Unsplash
1They don’t seem to get their meaning by contrast with other words either. Certainly, NLP systems, and, at a guess, our brains, don’t actually do the explicit act of differance – what we do is calculate similarity and extract the nearest neighbors both for other sentences and for memories (including visual ones). That does not mean that it is impossible to create an interpretation of similarity search in terms of vector dissimilarity.
2This paragraph refers to the act of actually writing down the words. However, this is meant only to clarify the possibility of divorce of the written sign from the mental associations present whenever we speak or think these sentences. In practice, once the proposal has been clarified in this way, there is no reason not to suggest a parallel distinction being formed in a thinker’s head too.
Comments